AImpotence: When Your AI Just Can’t Deliver the Goods, the Issue Might Lie in What You’re Feeding It
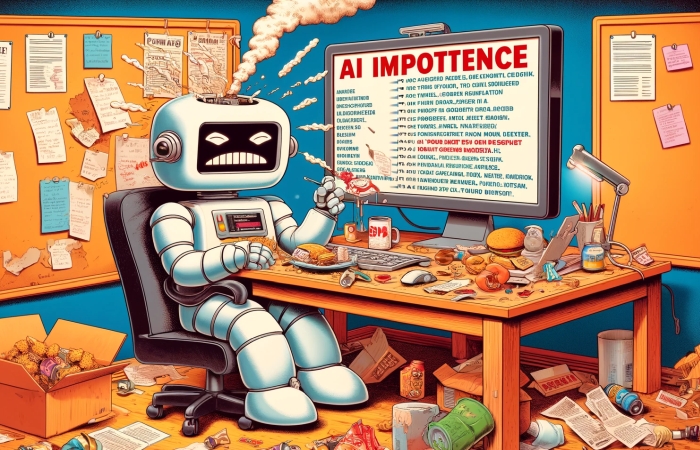
AI is changing the world, but true Business-ready AI is still rare.
The secret to getting the most from your AI, like Microsoft Copilot, is to present it with a library of data that has structure and meaning.
Computer One can spearhead a Data for AI project.
OpenAI took the whole world and threw it into a new reality in the last months of 2022 with the public release of ChatGPT. And in doing so, the Microsoft-backed company kicked off one of the most all-encompassing, all-consuming technology races the world has ever seen.
Suddenly, if your tech product didn’t have an association with AI, your brand was immediately less relevant. And so, the world of developers, product engineers and marketers alike has been consumed since in trying to drive a meaningful connection to AI.
Most efforts are just a passing association. Adding AI can be as simple as tacking on a backend link to ChatGPT or another large language model to output text responses in some way. Lip service to the notion of true artificial intelligence.
A few, like Microsoft’s Copilot, might actually evolve to deliver on the real promise of AI: not just conversation, but intelligent, productive action.
There’s just one (large) problem. Data.
To become useful to your organisation, at a level beyond simply helping to format text, your AI has to really understand you: what your organisation does, how your documents are formatted, how you communicate in writing, etc. In many respects, this is like an induction and orientation program for a new employee – you have to show them the norms of your organisation, the assets at hand and the constraints within which they will have to work.
This is the part that AI integration in workplaces is stuck on, worldwide.
Because without that induction, or “ingestion”, as data experts call it, Copilot and other AI tools can’t be expected to deliver results that really move the needle on enhanced productivity. In simple terms, if you’re not feeding your AI correctly and training it on what productive results look like, it’s going to struggle to deliver value.
So, the first step in embracing AI, doesn’t actually involve AI at all. It is to look inwards and improve the quality of the input you’re going to use to train it. Locating, identifying and enriching your data is key.
This is a problem for many organisations, whose data is spread between email repositories, user desktops, network drives, Teams folders and SharePoint implementations. Knowledge is unstructured, file-level meta data is not applied and correct contextual understanding can’t be achieved.
First the data must be located, then it must be transformed and/or brought into a common repository and structured beneficially for your AI implementation. Then, it must be vetted for sensitive information. Why? Because data security is often not applied correctly to file repositories. And so, your AI can occasionally access sensitive information that it goes on to share with unauthorised users. This is not an outcome you want.
Only then will your data be ready for your AI to consume, break-down and turn into useful contextually-correct output when you interact with it.
How do you tackle this data project at scale? You will undoubtedly find benefit in a programmatic solution. Computer One and companies like ours can write scripts to identify, categorise and transform data to provide structure and context for your AI. Or there are several off-the-shelf applications that can be utilised. Every organisation is different and the solution to your Data for AI project is going to be uniquely yours.
One way or another, to get the most from AI, you’re likely to need a Data for AI project like we have described. Let’s talk about what that project might look like for your business, with no obligation attached.
Call us on 1300 667 871 or chat to us live on site.